Soft sensors: what you get when you combine profound knowledge of industrial processes with a deep understanding of modern AI tools
What’s a soft sensor?
A soft sensor is an inferential estimator when a hardware sensor is unreliable or unavailable. The soft sensor is used for operator decision support, maintenance, and control purposes. The other application of a soft sensor is to replace or support an expensive analyser that needs more frequent calibration, consequently reducing the cost. Soft sensors, like other online analysers, need frequent calibration. However, unlike most hardware sensors, the calibration can be done remotely and operations don’t need to have the technology provider onsite to calibrate and service the instrument, saving cost and time, which is particularly important for remote operations. While conventional soft sensors were mainly based on linear and physical models, modern machine learning techniques bring opportunity to improving the commonly used soft sensors and provide new types of soft sensors that couldn’t be developed through conventional methods.
Developing an advanced soft sensor requires a combination of profound knowledge of the process, challenges, and opportunities along with a deep understanding of modern AI tools. This skillset can be provided through an integrated team of process engineers, control experts, and data scientists.
Deploying an advanced soft sensor is not a free-risk solution. Relying only on data-driven tools to develop a soft sensor leads to unforeseen challenges if the data doesn’t cover all operation conditions. Furthermore, a perfect database with the proper size and granularity doesn’t exist in most cases. Therefore, subject matter experts’ (SMEs) involvement is crucial to help data scientists in all development stages from defining the problem and finding and/or building a useful database, to developing the model, testing, and deploying the solution.
How does collaboration happen? Here’s a step-by-step look
1. It starts with problem definition. Data scientists and SMEs need to come out with the same language to define the project in understandable terms for all stakeholders. Data scientists should understand what the need for a soft sensor is and how a user can apply it to infer a KPI. On the other hand, SMEs need to understand the methodology for developing a soft sensor in order to provide the right input. Once both parties achieve a mutual understanding, they need to break down the project into scopes and define a measure of success. The measure of success is a function of the accuracy and reliability of the soft sensor.
2. Next comes the data preparation and understanding the relationship between variables, delay, bias, measurement error, etc. Knowing the relationship between variables is required to prevent misleading data scientists and selecting the right modeling tools in the next step.
3. Which brings us to the next step: modeling. Depending on what modeling approach is selected, SMEs will have different input. For example, in developing deep learning-based soft sensors, SMEs can help define different inducive knowledge-based biases (a set of assumptions to predict outputs). However, the main contribution can be in hybrid modeling, which is the physical model combined with machine learning models. SMEs can develop the physical model or lead the team to use proper physical models. There are several types of hybrid modeling. These models can be classified into the following categories:
· Supplement: one can correct the other.
· Embedded: one model can be embedded in the other.
· Proxy: one used as a proxy for the other.
· Complement: the solution can be the combination of both.
· Integrate output: one model output can serve as an input of another.
· Structure mimic: one model can be drawn from another.
4. Data scientists have statistical metrics to validate model performance. However, selecting the right metrics needs to be confirmed by SMEs. Moreover, SMEs can validate the machine learning or hybrid model results with other tools and metrics such as simulation. At this stage, the team should decide whether model performance met the requirements in terms of accuracy and reliability or not. Then they can decide to move forward and deploy the solution or loop back and improve the model.
5. Deploying the solution is the next step and requires extensive effort and other stakeholder involvement. Data accessibility, compatibility, and security, along with system architecture, solution location, user experience, reporting, and defined responding procedures should be considered in this stage.
6. Lastly, calibration and support: like other sensors, soft sensor calibration is required to be done through autocalibration methods or by the SMEs. An advanced soft sensor relies heavily on data. As a result, rebuilding the model is required in some cases, particularly when the process significantly changes and the historian data doesn’t represent the current conditions.
In the spirit of innovating in all that we do, the industry should be taking advantage of advanced soft sensors in order to overcome the inadequate visibility of main KPIs and further use it as a tool for decision-making support.
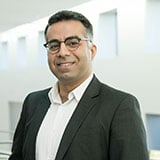
Ali Vazirizadeh
Global Practice Lead, Data Science
Ali has over fifteen years of experience in research and engineering, specializing in artificial intelligence and machine learning. He brings in-depth knowledge of data science, technology, and innovative solutions development through design and optimization projects, consulting, and research.